Backdoors in Machine Learning Models
Interest in machine learning has grown incredibly quickly over the past 20 years due to major advances in speech recognition and automatic text translation. Recent developments (such as generating text and images, as well as solving mathematical problems) have shown the potential of learning systems. Because of these advances, machine learning is also increasingly used in safety-critical applications. In autonomous driving, for example, or in access systems that evaluate biometric characteristics. Machine learning is never error-free, however, and wrong decisions can sometimes lead to life-threatening situations. The limitations of machine learning are very well known and are usually taken into account when developing and integrating machine learning models. For a long time, however, less attention has been paid to what happens when someone tries to manipulate the model intentionally.
Adversarial Examples
Experts have raised the alarm about the possibility of adversarial examples [1] – specifically manipulated images that can fool even state-of-the-art image recognition systems (Figure 1). In the most dangerous case, people cannot even perceive a difference between the adversarial example and the original image from which it was computed. The model correctly identifies the original, but it fails to correctly classify the adversial example. Even the category in which you want the adversial example to be erroneously classified can be predetermined. Developments [2] in adversarial examples have shown that you can also manipulate the texture of objects in our reality such that a model misclassifies the manipulated objects – even when viewed from different directions and distances.
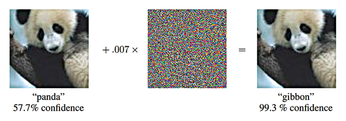
[...]
Buy this article as PDF
(incl. VAT)
Buy Linux Magazine
Subscribe to our Linux Newsletters
Find Linux and Open Source Jobs
Subscribe to our ADMIN Newsletters
Support Our Work
Linux Magazine content is made possible with support from readers like you. Please consider contributing when you’ve found an article to be beneficial.
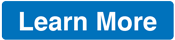
News
-
Linux Kernel 6.17 Drops bcachefs
After a clash over some late fixes and disagreements between bcachefs's lead developer and Linus Torvalds, bachefs is out.
-
ONLYOFFICE v9 Embraces AI
Like nearly all office suites on the market (except LibreOffice), ONLYOFFICE has decided to go the AI route.
-
Two Local Privilege Escalation Flaws Discovered in Linux
Qualys researchers have discovered two local privilege escalation vulnerabilities that allow hackers to gain root privileges on major Linux distributions.
-
New TUXEDO InfinityBook Pro Powered by AMD Ryzen AI 300
The TUXEDO InfinityBook Pro 14 Gen10 offers serious power that is ready for your business, development, or entertainment needs.
-
Danish Ministry of Digital Affairs Transitions to Linux
Another major organization has decided to kick Microsoft Windows and Office to the curb in favor of Linux.
-
Linux Mint 20 Reaches EOL
With Linux Mint 20 at its end of life, the time has arrived to upgrade to Linux Mint 22.
-
TuxCare Announces Support for AlmaLinux 9.2
Thanks to TuxCare, AlmaLinux 9.2 (and soon version 9.6) now enjoys years of ongoing patching and compliance.
-
Go-Based Botnet Attacking IoT Devices
Using an SSH credential brute-force attack, the Go-based PumaBot is exploiting IoT devices everywhere.
-
Plasma 6.5 Promises Better Memory Optimization
With the stable Plasma 6.4 on the horizon, KDE has a few new tricks up its sleeve for Plasma 6.5.
-
KaOS 2025.05 Officially Qt5 Free
If you're a fan of independent Linux distributions, the team behind KaOS is proud to announce the latest iteration that includes kernel 6.14 and KDE's Plasma 6.3.5.