Big Data, Python, and the future of security
Good vs. Bad
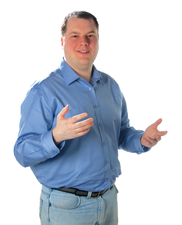
When you start processing security-related data to find patterns, you quickly end up in Big Data territory, and you'll need some powerful tools to help you separate the good from the bad.
Intrusion detection and prevention is a difficult problem, much like email spam. Basically, you want to block all the "bad" traffic without blocking any "good" data. Because you can't accomplish this perfectly, you have to make a choice of how much bad traffic you're willing to allow, and how much good traffic you're willing to block.
Generally, people take one of three positions here. The first is the infamous "we can't block any good traffic, we'll lose sales, etc." The second approach is "I don't care about inconveniencing anybody, block by default and make sure anything coming through is good." The third option is a little more subtle and difficult to implement; basically, you turn to economics and try to figure out the cost of blocking good traffic (annoying users, support costs) and the cost of not blocking bad traffic (cleaning up after the occasional intrusion), and you make a decision. The third option, however, is rarely based on actual data and is mostly done along the lines of "how much can we annoy users before they yell at us." But, it's better than nothing.
Big Data Tools
Processing all this information, of course, leads to Big Data. Personally, I'm not a fan of buzzwords, but enough incremental change usually leads to entirely new things. Today, I was backing up an email account that contains messages about the size of my first hard drive, and the entire mailbox was larger than the storage of my first seven or eight computers put together. The reality is, if you want to start processing security-related data to find patterns, you're going to end up in Big Data territory quite quickly.
[...]
Buy this article as PDF
(incl. VAT)