Finding problems using unsupervised image categorization
Needle in a Haystack
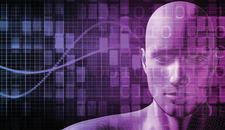
The most tedious part of supervised machine learning is providing sufficient supervision. However, if the samples come from a restricted sample space, unsupervised learning might be fine for the task.
In any classification project, it is certainly possible to get someone to review a certain number of images and build a classification list. However, when entering a new domain, it can be difficult to identify domain knowledge experts or to develop a ground truth for classification upon which all experts can agree. This is true regardless of whether you are looking at the backside of a silicon wafer for the first time, or if you are trying to identify the presence of volcanoes in radar images from the surface of Venus [1].
Alternatively, you can bypass all these problems and kick-start a classification project with unsupervised machine learning. Unsupervised machine learning is particularly applicable to environments where the typical images are largely identical, much like the pieces of hay in the haystack that you need to ignore when looking for needles.
In this article, I examine the potential for using unsupervised machine learning in Python (version 3.8.3 64-bit) to identify image categories for a restricted image space without resorting to training neural networks. This technique follows from the long tradition within engineering of finding the simplest solution to a problem. In this particular case, the solution relies upon the ability of the functions within the OpenCV and mahotas computer vision libraries to generate parameters for the texture and form within an image.
[...]
Buy this article as PDF
(incl. VAT)