A tour of some important data science techniques
Method in the Madness
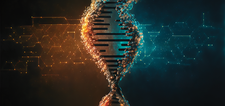
Data science is all about gaining insights from mountains of data. We tour some important tools for the trade.
Data is the new oil, and data science is the new refinery. Increasing volumes of data are being collected, by websites, retail chains, and heavy industry, and that data is available to data scientists. Their task is to gain new insights from this data while automating processes and helping people make decisions [1]. The details for how they coax real, usable knowledge from these mountains of data can vary greatly depending on the business and the nature of the information. But many of the mathematical tools they use are quite independent of the data type. This article introduces you to some of the methods data scientists use to squeeze insights from a sea of numbers.
More than Just Modeling
The term data scientist evokes associations with math nerds, but data science consists of far more than building and optimizing models. First and foremost, it involves understanding a problem and its context.
For example, imagine a bank wants to use an algorithm to predict the probability that a borrower will be able to repay a loan. A data scientist will first want to understand how lending has worked so far and what data has been collected in this field – as well as whether that data is actually available – with a view to data protection requirements. In addition, data scientists need to be able to communicate their findings. Storytelling is more useful than presenting infinite rows of numbers, because the audience is likely to be made up of non-mathematicians. The need to clearly explain the findings frequently presents a challenge for less extroverted data scientists.
[...]
Buy this article as PDF
(incl. VAT)