A tour of some important data science techniques
Method in the Madness
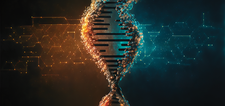
Data science is all about gaining insights from mountains of data. We tour some important tools for the trade.
Data is the new oil, and data science is the new refinery. Increasing volumes of data are being collected, by websites, retail chains, and heavy industry, and that data is available to data scientists. Their task is to gain new insights from this data while automating processes and helping people make decisions [1]. The details for how they coax real, usable knowledge from these mountains of data can vary greatly depending on the business and the nature of the information. But many of the mathematical tools they use are quite independent of the data type. This article introduces you to some of the methods data scientists use to squeeze insights from a sea of numbers.
More than Just Modeling
The term data scientist evokes associations with math nerds, but data science consists of far more than building and optimizing models. First and foremost, it involves understanding a problem and its context.
For example, imagine a bank wants to use an algorithm to predict the probability that a borrower will be able to repay a loan. A data scientist will first want to understand how lending has worked so far and what data has been collected in this field – as well as whether that data is actually available – with a view to data protection requirements. In addition, data scientists need to be able to communicate their findings. Storytelling is more useful than presenting infinite rows of numbers, because the audience is likely to be made up of non-mathematicians. The need to clearly explain the findings frequently presents a challenge for less extroverted data scientists.
[...]
Buy this article as PDF
(incl. VAT)
Buy Linux Magazine
Subscribe to our Linux Newsletters
Find Linux and Open Source Jobs
Subscribe to our ADMIN Newsletters
Support Our Work
Linux Magazine content is made possible with support from readers like you. Please consider contributing when you’ve found an article to be beneficial.
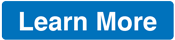
News
-
Red Hat Releases RHEL 10 Early
Red Hat quietly rolled out the official release of RHEL 10.0 a bit early.
-
openSUSE Joins End of 10
openSUSE has decided to not only join the End of 10 movement but it also will no longer support the Deepin Desktop Environment.
-
New Version of Flatpak Released
Flatpak 1.16.1 is now available as the latest, stable version with various improvements.
-
IBM Announces Powerhouse Linux Server
IBM has unleashed a seriously powerful Linux server with the LinuxONE Emperor 5.
-
Plasma Ends LTS Releases
The KDE Plasma development team is doing away with the LTS releases for a good reason.
-
Arch Linux Available for Windows Subsystem for Linux
If you've ever wanted to use a rolling release distribution with WSL, now's your chance.
-
System76 Releases COSMIC Alpha 7
With scores of bug fixes and a really cool workspaces feature, COSMIC is looking to soon migrate from alpha to beta.
-
OpenMandriva Lx 6.0 Available for Installation
The latest release of OpenMandriva has arrived with a new kernel, an updated Plasma desktop, and a server edition.
-
TrueNAS 25.04 Arrives with Thousands of Changes
One of the most popular Linux-based NAS solutions has rolled out the latest edition, based on Ubuntu 25.04.
-
Fedora 42 Available with Two New Spins
The latest release from the Fedora Project includes the usual updates, a new kernel, an official KDE Plasma spin, and a new System76 spin.